The Challenges and Opportunities of AI for Additive Manufacturing
Keynote panel at the DE Design & Simulation Summit explores the potential for artificial intelligence to enhance design for additive.
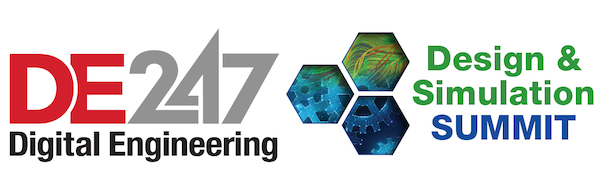
Latest News
October 27, 2023
Artificial intelligence (AI) and machine learning (ML) can potentially help engineers streamline and improve their design for additive manufacturing (DfAM) efforts, but leveraging AI in these applications will require enormous amounts of data and careful thought about where and how to deploy the technology. Those were some of the thoughts presented at the keynote session for the Design & Simulation Summit earlier this week.
The Design & Simulation Summit, presented by Digital Engineering, kicked off at 1 pm Eastern on Oct. 26 with a keynote panel titled What Can AI Bring to Design for Additive Manufacturing? moderated by Senior Editor Kenneth Wong.
Panelists included:
- Dr. Yaoyao Fiona Zhao, Associate Professor and William Dawson Scholar, Department of Mechanical Engineering, McGill University. Her lab has developed a number of software tools that leverage AI for different aspects of design.
- Matthew Shomper, Founder of LatticeRobot.
- Chris McComb, Faculty Member at Carnegie Mellon University’s Department of Mechanical Engineering.
A key piece piece of the AI puzzle moving forward will be accumulation and aggregation of geometric and other types of data to train AI models. “Every AI solution depends on a ton of supporting infrastructure – data, the storage infrastructure, data cleaning, analytics and more,” McComb said. “We need to care a lot about that data infrastructure.”
With powerful pattern recognition and natural language processing, AI or machine learning is expected to drastically lower the learning curve for design and simulation software and transform its user experience. Many leading design and simulation software companies are already incorporating AI into their software offerings.
Additive manufacturing presents additional challenges in design, since the technology allows for the creation of new types of shapes and structures not possible with traditional manufacturing approaches. That means the design space is much larger, and 3D printers can utilize different types of materials with different properties. Can AI improve DfAM (Design for Additive Manufacturing) in the same way as it does for traditional design? Can AI give existing DfAM algorithms a boost in failure detection and geometry generation?
According to the panelists, there are ways to use AI to improve DfAM, but there are also many challenges.
Shomper said that engineers have been limited in their ability to apply multifunctional, multiscale design techniques because of the complexity of the problems these designs pose, and as well as difficulties in communicating design information.
“I think one of the huge ways we can leverage machine learning in design for additive is with CAD tools,” he said. “That is where we need to take this.”
Dr. Zhao noted that a lot of design knowledge, graphics, modeling information, and more are deeply embedded in design processes, but it is difficult to extract useful data.
“This is where AI and ML can play a role, but that has not gained a lot of research attention yet,” she said. “Generative AI research for topology optimization has gained attention, but topology optimization is only part of the overall geometric modeling effort that supports additive manufacturing.”
McComb also noted that while large language models can easily access data sets for algorithm training, the same is not true for design work. “In a lot of cases we create the data ourselves, by measuring it from real world design projects, working with companies to identify the data they have, or synthetically generating that data,” McCom said. “For additive manufacturing, that is a bit of a problem, because for a lot of processes we have some simulations that provide good results, but not enough to really make good design decisions yet. And not all data is created equal.”
Leading Edge Design Presentations
In addition to the keynote, the Summit also included the following sessions:
Mark Davies, director and owner of HAAR Technology in the UK, presented Digitization and Robust Requirement Writing for Procurement Standardization in the Oil and Gas Sector, which provided an overview of the value of standardization and clarity in requirements and specifications, through the lens of new procurement standards in the oil and gas industry.
In a presentation titled Engineering Analysis: The Good, the Bad and the Ugly, Ted Fryberger, president of DeepSoft, will provide tips for improving FEA and CFD workflows across a variety of tools and workflows.
Attendees learned how to select the right options – workstations, HPC, or cloud – for their specific workflows in a session titled The Future of Engineering Computing: From Workstations to the Cloud. MingYao Ding, principal and vice president of engineering at Ozen Engineering, explained how GPUs have revolutionized simulation performance, and what options are available in terms of workstations and cloud solutions for accelerating these workflows.
Attendees learned from TRAK Machine Tools how the company was able to deploy digital twin technology to improve manufacturing operations. Tom Copeland, vice president of operations and chief technology officer (CTO) at TRAK presented a case study explaining how small and medium-sized businesses can deploy and leverage the benefits of digital twins.
All presentations are now available on demand at the event website.
Subscribe to our FREE magazine,
FREE email newsletters or both!Latest News
About the Author
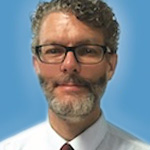
Brian Albright is the editorial director of Digital Engineering. Contact him at de-editors@digitaleng.news.
Follow DE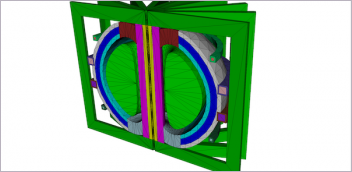
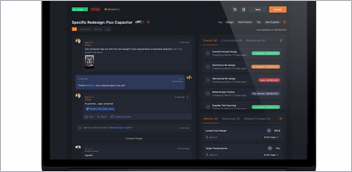
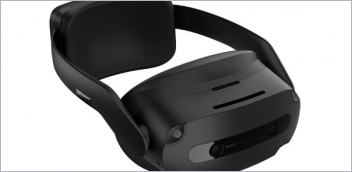
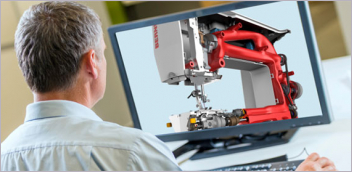