What Will Digital Twins Look Like in 5 Years?
A clear definition and view of the technology is only now taking shape.

A meshed network of digital twins may entail twins of a residential building and a commercial building, as well as a twin integrating these and other twins to create a smart city model to predict energy use and carbon production. Image courtesy of Siemens Digital Industries Software.
Latest News
December 8, 2023
Digital twin has been around for about 15 years, and you would think that by now it would have taken on a clear, well-defined definition. Unfortunately, you would be wrong—at least somewhat. The evolutionary path of this concept, the form of the technology and its application can best be characterized as a series of fits and starts. The fact is that companies are only just now approaching the point where they can begin to achieve the promise of digital twins.
The market has only recently begun to embrace a monolithic vision of the technology. Until now, the concept of digital twin meant different things to different people, with its definition shaped by the economic and technological limitations within which the implementer has had to work.
Not surprisingly, the future of digital twin promises to be as fractured as its past, with some market segments playing serious catch-up to the original vision while others stand poised to make incredible leaps forward (Fig. 1).
Off to a Slow Start
To conjure a realistic picture of digital twin’s future, you have to understand its past. Initially, leading proponents of the digital twin concept introduced an active technology that supported interaction between complex, expensive physical assets produced in volume, such as wind turbines and their digital twins.
Yet the initial vision of digital twin did not gain enough traction to support widespread adoption. This particular vision required more data-management resources than most organizations could provide cost-effectively. These companies simply could not cultivate or afford the infrastructure needed to supply contextualized data in an analysis-ready format.
“The economics here might work for something like a jet engine, but it didn’t work everywhere else,” says Kurt DeMaagd, chief artificial intelligence officer at Sight Machine. “Because of that, digital twins were redefined as passive digital replicas, 3D visualizations, 2D visualizations with some small degree of basic key performance indicators or other metrics associated with the visual.”
Steady growth and refinement of the technology since its introduction, however, has allowed the market to advance beyond the simpler conception of the digital twin. Since the early phases of digital twin implementation, the scope of the concept has expanded. It has evolved to become a more active representation, with bidirectional interaction between the physical entity and its digital twin, accompanied by an expanded digital thread and a feedback loop between the physical and digital worlds, allowing entity enhancement and optimization.
Taking digital twin technology to this level sets the stage for dramatic advances in physical-digital interaction and autonomous decision-making. That said, significant challenges remain. Overcoming these obstacles will require a number of emerging technologies to deliver critical enabling components for digital twin’s success.
“Achieving a comprehensive representation of reality—especially in a cost-effective manner—is proving to be challenging,” says Steve Dertien, chief technology officer at PTC.
The Trouble with Technology
As the scope of the digital twin concept expands, the challenges facing developers and implementers of the technology grow at an equal or greater rate. Some of these challenges have been inherent with digital twin since its introduction, while on the other hand, others have arisen as the concept of the technology has evolved.
As you would expect, data sits at the top of the list of technical challenges. Even with the growth of data storage and compute capability, there are still significant challenges associated with data.
“A key challenge is to capture the most relevant data required to detect, forecast or optimize at the right time and price point,” says Colin Parris, chief technology officer of GE Vernova’s Digital business. “This may require new sensor capabilities, embedded at the right locations and with the right capabilities.”
Another facet of the data challenge is the fusion, storage and management of the data, especially if the data is going to be used by artificial intelligence (AI) engines to provide enhanced insight.
“Handling and integrating vast amounts of data from diverse sources is a significant uphill battle,” says Camilla Tran, business development and ecosystem partner at Ericsson One. “The primary goal here is to ensure data quality, security and interoperability among various digital twin instances and their physical counterparts. When it comes to interoperability, different industries and systems use various standards and protocols. Here, developers must address the issue of interoperability to ensure that digital twins can communicate effectively across sectors, devices and platforms.”
Closely associated with the data challenges is the constant demand for more computing resources.
“Given the widespread use of AI technologies in the digital twin, there will be greater need for compute capability,” says Parris. “This is especially true in training and creating complex systems and models. These compute demands have grown rapidly with the increased attention on large language and foundational models, and the limitations of [graphics processing units (GPUs)] and other key chipsets will pose challenges that will only get worse as time goes on.”
Looking at the broader picture, you cannot discuss data and computing resource challenges without considering the difficulties that arise when integrating emerging technologies into digital twins.
“Incorporating emerging technologies like AI, IoT [Internet of Things} and edge computing seamlessly into digital twin ecosystems is complex,” says Tran. “Ensuring that these technologies work together cohesively and efficiently is a significant undertaking because the ecosystem is so big. Furthermore, making sure connected objects also work together to give more than the sum of the parts is where the major challenges are: Namely, making different solutions not designed to work together perform a new common task.”
The Human Side of the Equation
On the human side, one of the greatest hurdles facing the next generation of digital twin implementers is the shortage of engineers that have adequate domain and AI skills.
“To create a useful digital twin, a company must have deep domain knowledge to determine what insight is required and what methods should be used to produce value,” says Parris. “It also requires the practitioner to understand the AI capability to determine the best models and the limitations of each so that validity and verification can be preserved—especially given the fast pace of AI’s evolution.”
Unfortunately, it’s difficult to find these skills, so they must be grown in the respective domains by key companies doing actual work and getting good results. Ultimately, the question becomes: Can the technology leaders in this sector keep pace with the demand for skilled practitioners? Unfortunately, the infusion of advanced technologies so essential to the continued growth of digital twin also exacerbates the skill shortage.
AI Is the Digital Twin’s Springboard to the Future
Prominent among the technologies proving to be double-edged swords, AI has carved out a key place in digital twin’s toolset, providing an essential ingredient for making advanced forms of the virtual technology a reality across various domains.
“Over the next 5 to 10 years, AI will play a major role as the digital twin evolves,” says Dale Tutt, vice president of industry strategy at Siemens Digital Industries Software. “AI already helps eliminate mundane tasks and improves computing efficiency, but I foresee it becoming a factor in automating the creation of digital twins and the connections between the 3D geometry and different simulation models that comprise it. AI will allow companies to take real-world data and identify the best way to drive that learning back into the digital twin to be used more effectively.”
The AI that will play these roles will not be the same technology companies are using today. Rather, it will likely be a new and improved version that does not have the shortcomings of today’s AI systems.
For example, digital twins are usually the combination of physical and data-driven models, many of which are now AI models and convoluted neural nets for visual analysis, recurring neural nets for time-series analysis or generative AI, such as large language models [LLMs] for text analysis. These AI models are often viewed as “black boxes” that give an outcome but do not explain how they arrived at the response.
In the past few years, however, technologies have emerged that promise to help to explain the decisions that the AI systems make. A good example of this are physics-informed neural nets, which use the laws of physics that pertain to the relevant domain to train the model and limit the space of admissible solutions. This gives more confidence in the use of the digital twin and reduces the business risk.
“An example of causal AI would be the use of an LLM in a Q&A mode to increase the productivity of field techs,” says Parris. “There are usually many manuals and a lot of update documentation pertaining to complex assets or systems in the field. An LLM—such as a secure enterprise ChatGPT—can be used to work with the field tech to help diagnose problems and provide recommendations for next steps. An example would be a question like: ‘What are the top five situations or statuses that would cause ALERT 721-4, and what actions should be taken to indicate which is the actual situation?’ The LLM should provide the needed response as well the actual references—the appropriate manual and update documentation by manual ID, page number and confidence ranking.”
Fusing Physics and Data
AI technology in the form of machine learning is also being brought to bear in a new digital twin concept called hybrid digital twin (Fig. 2). This approach takes aim at improving the accuracy of simulations and enhancing digital twin’s ability to monitor evolving real-life conditions.
Companies can develop this type of twin using hybrid analytics, a set of machine learning tools that facilitate the combining and use of I/O from physics-based and data sensor–driven models. This approach eliminates, or at least mitigates, the shortcomings of each of the types of models.
For example, physics-based simulation models make excellent digital twins when the underlying physics of an asset are well understood and can be modeled with known equations. These models, however, represent idealized versions of the asset and cannot emulate real-life conditions well. As a result, they deviate from reality with margins of error that can be significant, and their ability to deal with large, complex systems is often compromised.
Hybrid digital twins counter this shortcoming by adding data-driven models to the mix. Sensor data from smart, connected products provide up-to-date insight into behaviors. Hybrid analytics then help physics-based simulations learn from the real-world data and move closer toward an accurate perception of real-life conditions, increasing the accuracy of simulations. This improves the digital twin’s ability to study large systems of systems, which would be problematic using physics-based models alone.
This new digital twin is positioned to significantly enhance design and product development. The potential accuracy and flexibility of the approach promises to increase users’ confidence in the insights provided by digital twin and to broaden adoption.
“We expect more usage of hybrid analytics techniques,” says Sameer Kher, senior director of product development, systems and digital twins, at Ansys. “Data-based twins have been the starting point, but they are increasingly falling short. Hybrid digital twin refers to the use of models that combine physics-based models, which incorporate domain knowledge, with data-driven machine learning, which allows the model to evolve, accounting for factors such as operational differences and wear and tear.”
Future Digital Twin Architectures
The next evolution of the digital twin concept resides just over the horizon. These concepts aim to leverage advances in sensor technologies, AI and IoT systems to contend with increasing complexity and the broadening scale of systems of systems. Unlike hybrid digital twins, these technologies are still in the conceptual stage.
The first of these constructs is the self-generating digital twin, which would be capable of autonomously creating and updating its digital representations without human intervention. These digital twins would continuously collect and analyze data from their physical counterparts and adapt their models and parameters to reflect real-world changes, using AI and machine learning.
“The emergence of self-generating digital twins would be triggered by advances in sensor technology, AI and machine learning algorithms,” says Tran. “These technologies would enable digital twins to learn from and adapt to real-world data, self-generating based on new information and scenarios.”
The realization of this concept depends heavily on how much of the ecosystem (e.g., external sensors and connectivity technologies) is enabled. More and more digital twins connect to other data sources and have an AI layer, the primary key to enabling self-generating digital twins.
Another future digital twin architecture waiting in the wings is called hierarchies of digital twins. In these multi-tiered structures, digital twins represent components at various levels of complexity, ranging from individual sensors or components to subsystems and entire systems. In these constructs, information would flow up and down the hierarchy, allowing for detailed analysis and system-level optimization.
The driving force behind an introduction of hierarchies of digital twins would be the need for more comprehensive system insights and better control. As systems become increasingly complex, hierarchical digital twins would help manage the complexity.
Significant challenges, however, must be overcome before the hierarchies become practical.
“Currently, it is very difficult to integrate digital twins because they have different fidelities, scale and assumptions,” says Parris. “So there must be a way of standardizing these interfaces so that it becomes easy to link the output of one twin to the input of another and know that the result is accurate and valid.”
A third architecture being discussed is a meshed network of digital twins (see top image). In this construct, multiple digital twins would connect across different physical entities, creating a network of interlinked representations. The digital twins would exchange data, collaborate and collectively optimize processes or systems.
The factor driving the emergence of meshed networks of digital twins would be the desire for increased coordination and optimization across interconnected systems. This could be particularly relevant in smart cities, logistics and complex supply chains.
“The long-term evolution of the digital twin concept toward self-generating digital twins, hierarchies and meshed networks holds great potential for realizing ambient intelligence,” says Tran. “Advances in sensor technology, AI, communication protocols and data security are essential to making these mechanisms a reality and unlocking the benefits of ambient intelligence across various domains. It is just a matter of time until we reach there.”
More Ansys Coverage
More GE Digital Coverage
More PTC Coverage
More Siemens Digital Industries Software Coverage
Subscribe to our FREE magazine,
FREE email newsletters or both!Latest News
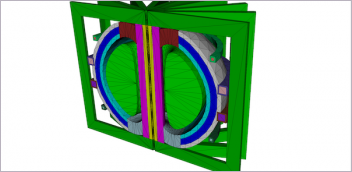
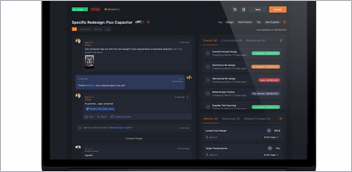
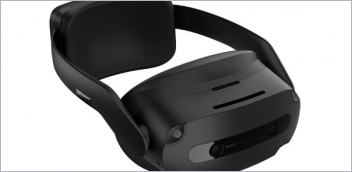
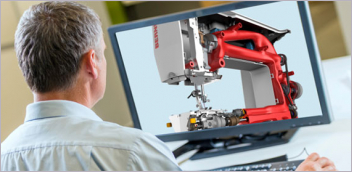