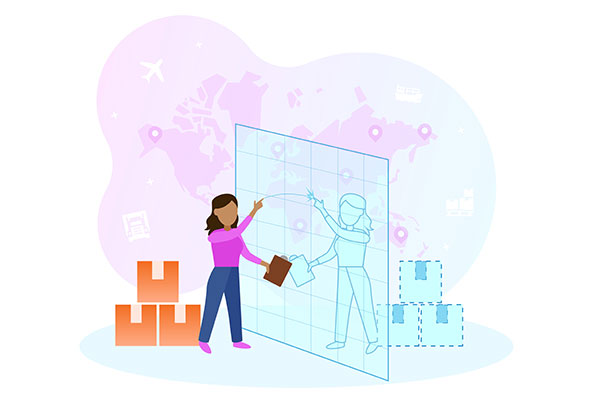
Fig. 1: Digital twins provide companies with the ability to model and simulate various supply chain scenarios—such as disruptions or changes in demand—allowing them to understand the potential impact on operations and to identify strategies for mitigating risks and improving performance. Image courtesy of Coupa.
Latest News
February 1, 2023
What has become painfully apparent in recent years is that there simply isn’t enough granularity of visibility or action across supply chains for companies to be effective when faced with significant and prolonged disruptions. There is, however, a solution to this dilemma, and it lies in emerging modeling and simulation capabilities enabled by digital twins.
The ability to model and simulate disruption across connected processes and to identify the critical nodes along the digital thread of a supply chain promises to deliver significant value for organizations (Fig. 1). Anomalies can be detected early, and notification events can be passed along the digital thread to up and downstream processes. Any impact on the master plan—good or bad—provides opportunity for action.
“With an integrated cross-functional model, an organization can synchronize preparations and extend the planning horizon across all supply chain processes, with the benefit of introducing flexibility into the supply chain,” says Andy Hancock, global vice president, Center of Excellence, SAP Digital Supply Chain. “Suppliers and logistics providers can be selected based on criteria not previously considered to be viable. Orders can be rerouted or redirected with minimal effort and with a known outcome.”
Context Becomes Essential
But model creation is easier said than done, and has a new set of requirements.
“The fundamental challenge of the data value chain is contextualizing structured and unstructured real-time data, whether it’s from physical assets; internal systems; external partner systems; or other external data regarding weather, traffic, or geo-political, social, or economic events,” says Prasad Satyavolu, North America engineering and manufacturing lead at Accenture Industry X.
This is where comprehensive digital twin cultivation comes into play. As the fidelity of the model increases and external entities of the ecosystem are included, the contextualized data opens the door for more effective modeling and simulation, ultimately providing a degree of control and decision-making power not previously available.
When Modeling Comes into Its Own
With an enriched supply chain data pool to draw from, digital twin-enabled modeling and simulation can have many valuable functions.
For example, armed with the right data, supply chain digital twins can support cost-to-serve analysis, modeling the end-to-end supply chain network and gleaning insights into the costs associated with manufacturing, transportation and storage. This analysis can also detail supply chain costs for delivering the products to customers.
Supply chain digital twins also enable demand modeling, using predictive analytics. This process identifies patterns, trends and anomalies in supply chain data, and makes predictions about future events and outcomes using artificial intelligence and machine learning techniques.
To address more immediate problems, digital twin modeling and simulation can perform control tower applications to manage near-term changes to supply chain operations (for example, changes to the route of a shipment or changes in the way goods are produced). In these cases, the goal is to improve efficiency and reduce costs. These applications rely on digital twins to validate their recommendations.
Supply chain digital twins also support within-four-walls simulations. These create virtual representations of supply chain assets, such as warehouses, factories and transportation networks, simulating and analyzing their performance and behavior using digital twin data. Discrete event simulation is a useful technology for modeling within-four-walls business scenarios.
The insights gleaned from these modeling and simulation applications provide benefits extending beyond those from more traditional technology.
“Organizations can make critical decisions closer to the event horizon and plan seemingly unrelated activities in parallel with full visibility of their interdependence and impact across the entire business, not just in the supply chain functions of the business,” says Hancock.
An example of this would be a company making a more informed and agile supplier selection by considering a supplier’s use capacity and its ability to meet service-level agreements rather than operating in a reactive fashion at the last minute..
Overall, business use cases addressed by supply chain digital twins vary from very long-term strategic business questions, such as capital planning, to short-term supply chain disruptions like the one mentioned already.
Looking Beyond the Expected
A valuable benefit of digital twin modeling and simulation is the capacity to analyze and compare results of numerous scenarios. These applications range from testing potential redesigns and changes to a supply chain to identifying and quantifying risks and evaluating the effects of supply chain disruptions.
One of the lessons that companies have learned from recent economic, political and social events is that supply chain designs must evolve to consider cost, speed, profit, customer service and risk. As the complexity of the supply chain increases, the ability to assimilate all the variables, recognize their relevance and identify possible solutions becomes exponentially more difficult.
This is where a digital twin scenario planning can assume the burden of complexity and elevate decision-making to a higher level. Here, modeling and simulation provide organizations with an opportunity to explore beyond the bounds of normality in the safety of a digital space, making it easier to identify bottlenecks, respond to anomalies, optimize inventory levels, understand the potential impact of decisions on operations and identify strategies for mitigating risks and improving performance.
At the moment of simulation, there is no guesswork required because companies have an exact digital copy of the current supply chain state. This brings some confidence to the results and recommendations.
As a result of increased visibility, companies gain a unified view of the supply chain, allowing decision makers to make qualifiable business decisions based on recommendations generated from a combination of real-time operational data, up-to-date business information and outputs from simulated “what-if” scenarios.
Hurdles Hindering Supply Chain Modeling
The chief factors that hinder current digital twin modeling and simulation lie in the rapidly growing number of factors that come into play in supply chain networks, the dynamic nature of their interactions, and the variety of conditions in which they operate.
“The limitation to achieving maximum potential performance lies in the exponential growth in complexity as the fidelity of the model increases and the constraints by one or more elements of time, cost or quality of the parties involved become a counterpoint,” says SAP’s Hancock. “The complexity manifests across dimensions that no one entity will have complete control over. Contract stipulations can coerce the immediate ecosystem to participate, but the value proposition and desire diminishes for participants the further away from the epicenter of the model they are.”
A deeper examination of the complexity highlights three subcategories of stumbling blocks: limited data sources, scalability and flexibility.
Digital twin technology relies on data to create virtual representations of supply chain processes and to monitor and analyze their performance. If data is not available or is of poor quality, it limits the accuracy and effectiveness of the digital twin model.
Compounding the problem, although digital twin technology is typically designed to handle large amounts of data, the size and complexity of a supply chain network can still pose challenges for some digital twin platforms. As a result, the system may not be able to effectively perform when dealing with very large or complex supply chains. Furthermore, the technology may not be flexible enough to adequately handle supply chain complexity.
“Digital twin technology is tailored to model and simulate specific supply chain processes and scenarios, but it may not be able to adapt to unexpected or unpredictable events,” says Nari Viswanathan, senior director of supply chain strategy at Coupa. “As a result, it may not be able to effectively respond to changes and disruptions in real time, unless it has been specifically designed and trained to do so.”
Value in Supply Chain Digital Twins
As expected with any new technology, there is a gap between implementing a supply chain digital twin and extracting the full value from the deployment. The factors responsible for the disparity fall into three categories: technological, organizational and human.
The technological challenge revolves around the digital twin’s need for quality data, specifically contextualized and compatible data. Providing an adequate supply of this kind of data has been hindered by the patchwork collection of systems and data sources that come into play.
“Digital twin technology typically involves the integration of multiple software and hardware systems and the sharing of data between these systems,” says Viswanathan. “This can be challenging, especially if the systems are not designed to work together or if there are compatibility issues. To overcome this challenge, companies can focus on implementing interoperable systems and technologies, and on using standards and protocols to facilitate data sharing and integration.”
The next two categories may ultimately prove to be the most challenging.
From the mid-20th century on, supply chain designs have aimed primarily to reduce costs. Decisions have been made based on where the labor is cheapest and what supplier comes in with the lowest bid. Recent events, however, have shown how inadequate this approach is in the face of significant disruptions. The resulting call for fundamental, structural change has been met with stiff institutional resistance.
“How is an organization going to reverse 20 years of globalization?” says Hancock. “It’s not easy to transform your supply chain from cost conscious to risk resistant and resilient. There will be a lot of ingrained processes that will be difficult to relinquish and may only be optimized.”
The organizational human-related challenges, however, go even deeper, with resistance occurring on the individual level.
“The human element will be at the heart of the challenges that will either make or break realizing the full value of a supply chain digital twin,” says Hancock, adding that the challenge will primarily stem from a lack of resource expertise in cross-topic areas. “Alignment of common functional goals and interoperability across engineering, planning, manufacturing, maintenance and logistics will be key.
“Do not underestimate the required level of trust,” Hancock adds. “People find it hard to give up control to an automated process or ownership of a controlling element. Change must be introduced not only as good for the business, but also in the evolution and betterment of work for the employee.”
In short, to extract full value from supply chain digital twins and the associated modeling and simulation capabilities, organizations and individual employees will have to embrace supply chain designs and processes that challenge existing notions.
Subscribe to our FREE magazine,
FREE email newsletters or both!Latest News
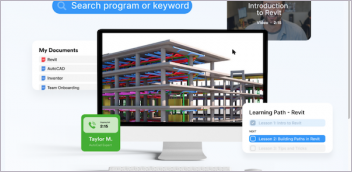
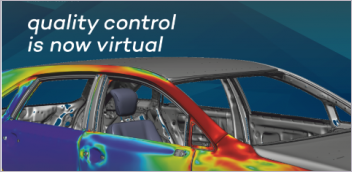
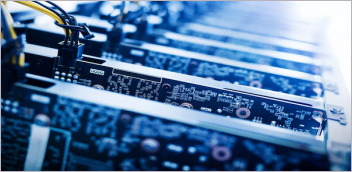
