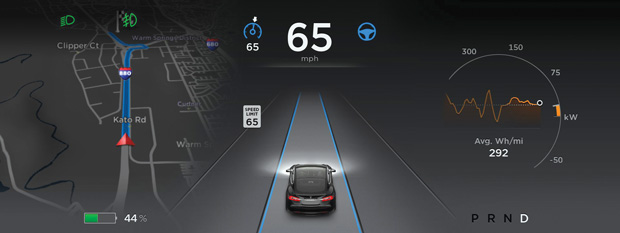
With self-driving cars, drivers can gather analytics about their route and conditions. Image courtesy of Tesla.
Latest News
August 1, 2016
In a comical YouTube video that has since been taken down, technologist Austin Meyer read aloud from a newspaper that blocked the entire view out the windshield of his Tesla Model S. The car is running on Autopilot, steering itself as well as keeping acceleration steady. But instead of demonstrating that hands-off control of self-driving cars is ready for prime time, Meyer was making the point that watching the road is still mandatory for safe driving, even in robotic cars. He in fact used a spotter in a pace car and drove on a sparsely traveled private road to pull off his newspaper stunt.
That’s because vehicles with autonomous capabilities still aren’t up to the task of everyday driving, as more recent and serious events have illustrated. On May 7, Joshua D. Brown died while driving his Tesla Model S in self-driving mode. Initial reports indicate that the car’s cameras could not distinguish the white side of a turning tractor-trailer rig from the sky, or may have identified it as an overhead sign, so the car’s brakes were not activated. The National Highway Transportation Safety Administration has launched an investigation.
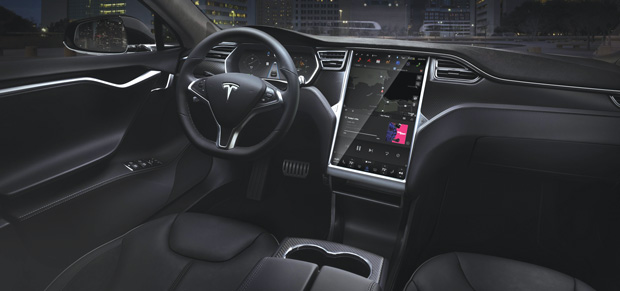
The crash has called attention to what those working on human-machine interaction already know: Much more work needs to be done to line up human expectations with fast-evolving robotic capabilities. The study of how to optimize human-robot interaction, or HRI, is a central challenge now facing both robot designers and users.
The Perception Problem
Perceptions, both human and robot, are at the heart of the HRI challenge. On the human side, says Sanjiv Singh, a research professor at Carnegie Mellon University’s Robotics Institute and CEO of drone startup Near Earth Autonomy, is a gap between the perceived abilities of robots like self-driving cars and their actual capabilities. “One of the dangers of automation,” says Singh, “is that people sometimes ascribe more intelligence to the automation than is really there.” And that can get people into trouble.
Singh cites a case where sponsors of an experimental autonomous lawn mower visited his lab at Carnegie Mellon to see a demonstration. After a few minutes of watching the mower, the sponsors grew comfortable enough with it to stop paying attention, even turning their backs to the golf-cart-size machine and its whirling blades as they excitedly discussed its potential. “We had to tell them,” recalls Singh, “this is a piece of heavy machinery that’s operating within feet of you. It could have an error.” The tendency is for humans to get too relaxed too quickly around potentially dangerous robots if they initially appear competent, which is something that Singh himself experienced while riding in an experimental autonomous car created by Google.
The limitation of software controlling autonomous vehicles, says Singh, is that although it may perform well in predictable conditions, it may not react so predictably—or at all—in unforeseen circumstances. For that reason a human has to keep a close watch and be ready to take over. And for a driver lulled into complacency, reaction may come too late to avoid an accident.
Thomas Sheridan, a professor emeritus of mechanical engineering at MIT, has studied human factors and HRI in a career going back to the 1950s, when he worked on airplane cockpit design in the U.S. Air Force. He cites studies showing that humans do not in fact react quickly enough to take over from misbehaving robots to prevent problems from occurring. “If there’s too little to do, a person just loses interest,” he says. This means human intervention won’t occur until it’s too late. “Keeping the human in the loop if the human’s got nothing to do is a virtual impossibility.”

Then, too, there is the problem of robot perception. “Right now we’re using lidar, cameras, radar. These are the three sensing modalities that are most in use,” explains Singh. All three are susceptible to errors caused by environmental changes. “A puddle on a road can create a complete black hole for a vehicle that’s driving using lidar,” for example, says Singh. “Water absorbs lidar and you will get no returns back from a puddle.”
Moving Toward Solutions
Sheridan suggests task sharing as a good principle for human-robot interaction because it keeps humans in the loop and interested enough in the task at hand to be ready to take control if necessary. Rather than take over completely, says Sheridan by way of example, “a smart cruise control very precisely takes care of the longitudinal control for you while you steer.”
Another advantage of task sharing, besides increased safety, is that it allows robotics developers to test their systems in the real world with fewer negative consequences. “It’s a good way to mature the technology because you get to learn over long periods of time what the failure modes are,” says Singh of systems that assist with the task of driving without taking control. “You can get started without actually having 100% reliability.”
Overall, says Sheridan, more research is needed to learn not only how robots behave, but how people interact with them as well. As he puts in in his paper, Human-Robot Interaction: Status and Challenges, “With regard to mental models, that is, what operators are thinking, what they know, whether they misunderstand, and so on, research is critical as systems get more complex and the stakes get higher.”
In the meantime, better catch up on your reading after you get home.
More Info
Subscribe to our FREE magazine,
FREE email newsletters or both!Latest News
About the Author

Michael Belfiore’s book The Department of Mad Scientists is the first to go behind the scenes at DARPA, the government agency that gave us the Internet. He writes about disruptive innovation for a variety of publications. Reach him via michaelbelfiore.com.
Follow DE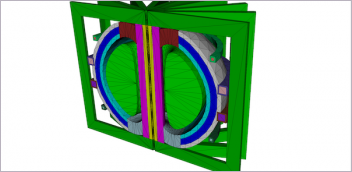
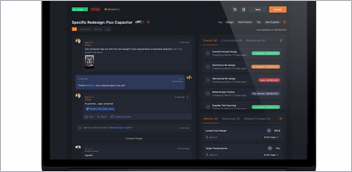
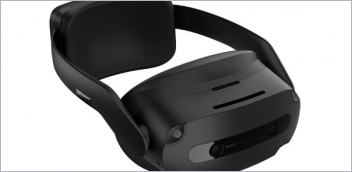
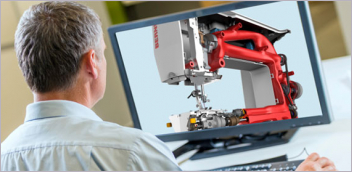